Research Highlights
Condition-Based Maintenance

CBM optimization
Condition monitoring variables can be used to improve predictions
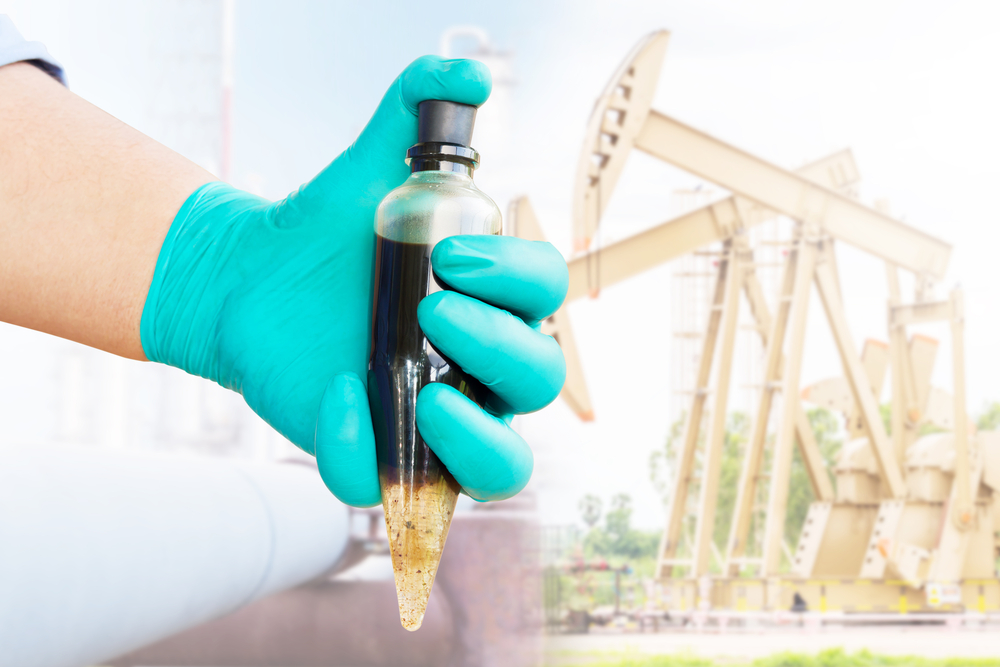
Measurement variable analysis
Many industrial assets are subject to large ranges of data collection. Which of these variables are worth measuring? Determine the statistically relevant measurement variables to predict equipment health.
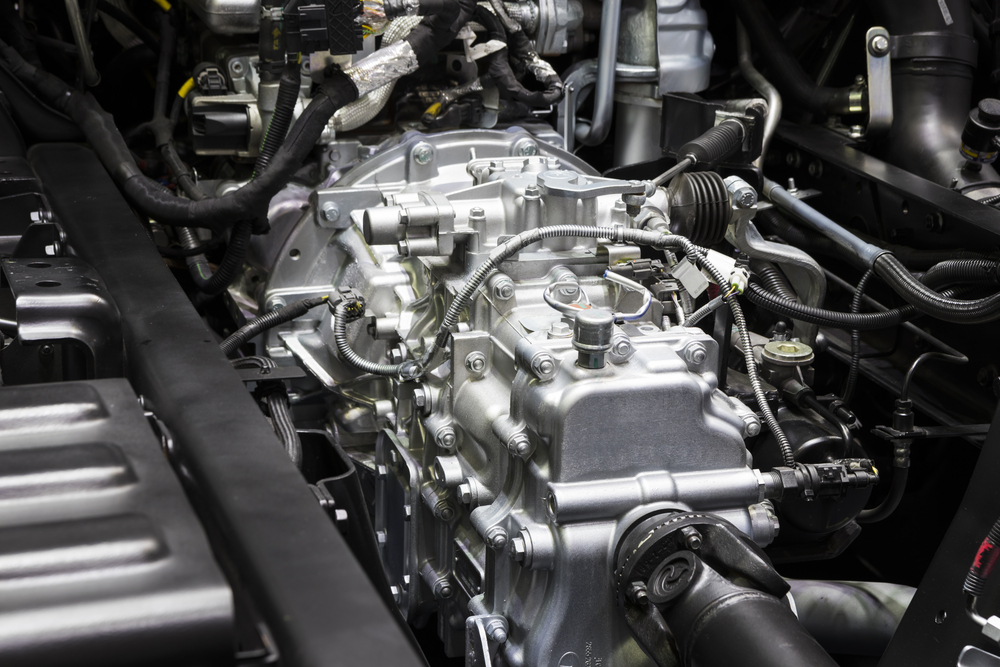
Component replacement decisions
When critical components age in large assets, its optimal replacement time may be considered. By combining condition monitoring data and economic information, make optimal decisions on when critical components ought to be replaced.
Asset Management
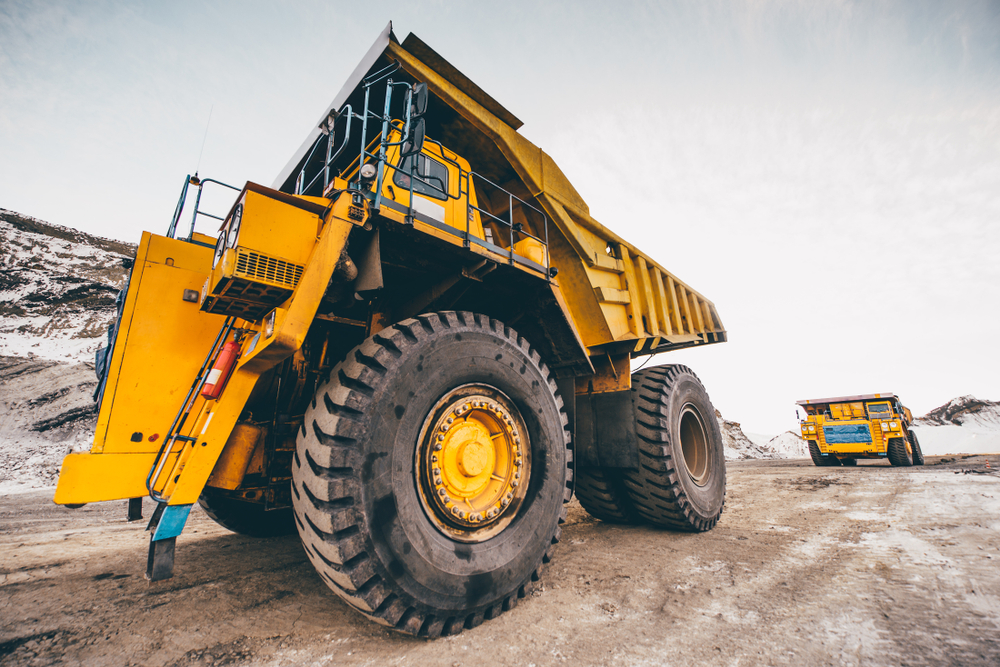
Life cycle costing
As assets age, they begin to spend more time in the shop being maintained and less time producing value for the company. Is there a sweet spot in which to replace the asset, even if it's still functioning?
Use life cycle costing to determine the value of an asset in each year.
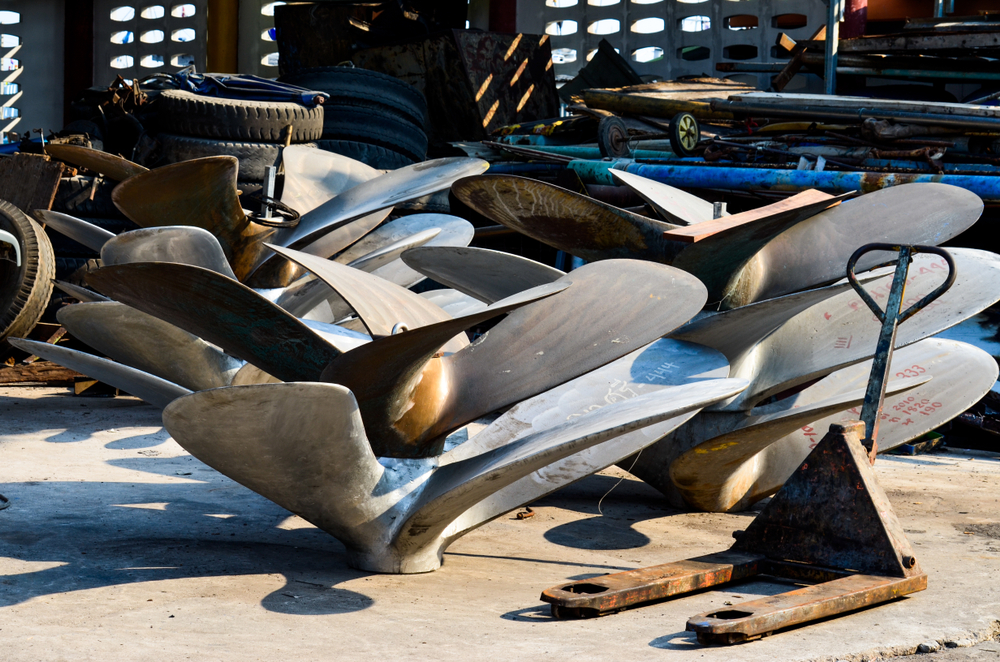
Spare parts analysis
When critical spare parts are large and expensive, it may not seem ideal to keep them in store. This leaves your organization at risk for a shut down when equipment fails. What's the right number of spares to stock when there's a long lead time, or the cost of downtime is very high?
Use our spare parts optimization software to maximize availability or minimize costs.
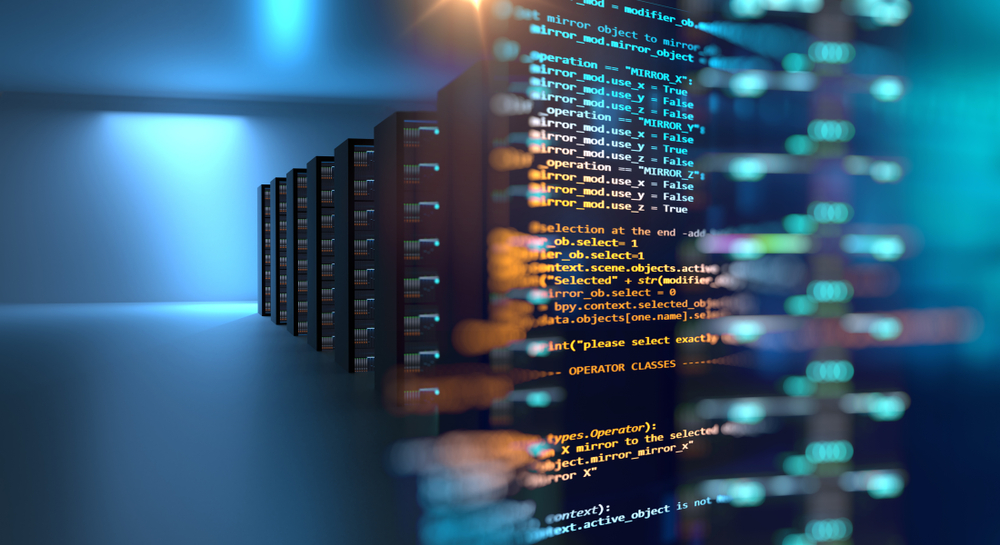
CMMS for reliability
The records currently being kept for maintenance management may require some modifications to be best suited for reliability analysis. We can advise on best practices for data warehousing and requirements for reliability engineering.
Machine Learning
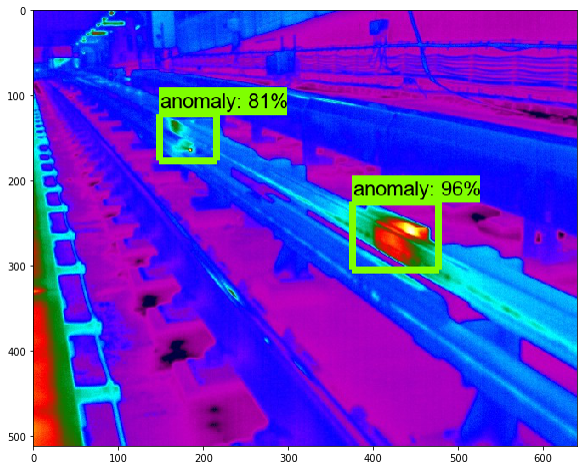
Anomaly detection
When there is a fault in your system, it can appear as an anomaly in your sensor readings. These faults or anomalies can be identified by trained individuals, but can take a lot of resources.
Use deep learning to automatically detect faults in your system.
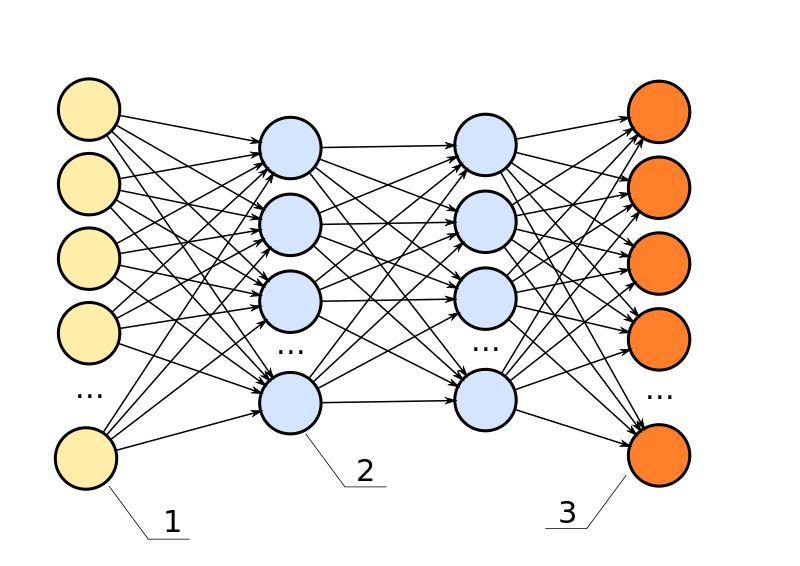
Neural networks for classification
An early-warning program may alert you to a defect, but knowing the precise nature of it will enable a targeted response. Use classification techniques powered by neural networks to automatically determine the category of defect based on the exhibited characteristics.
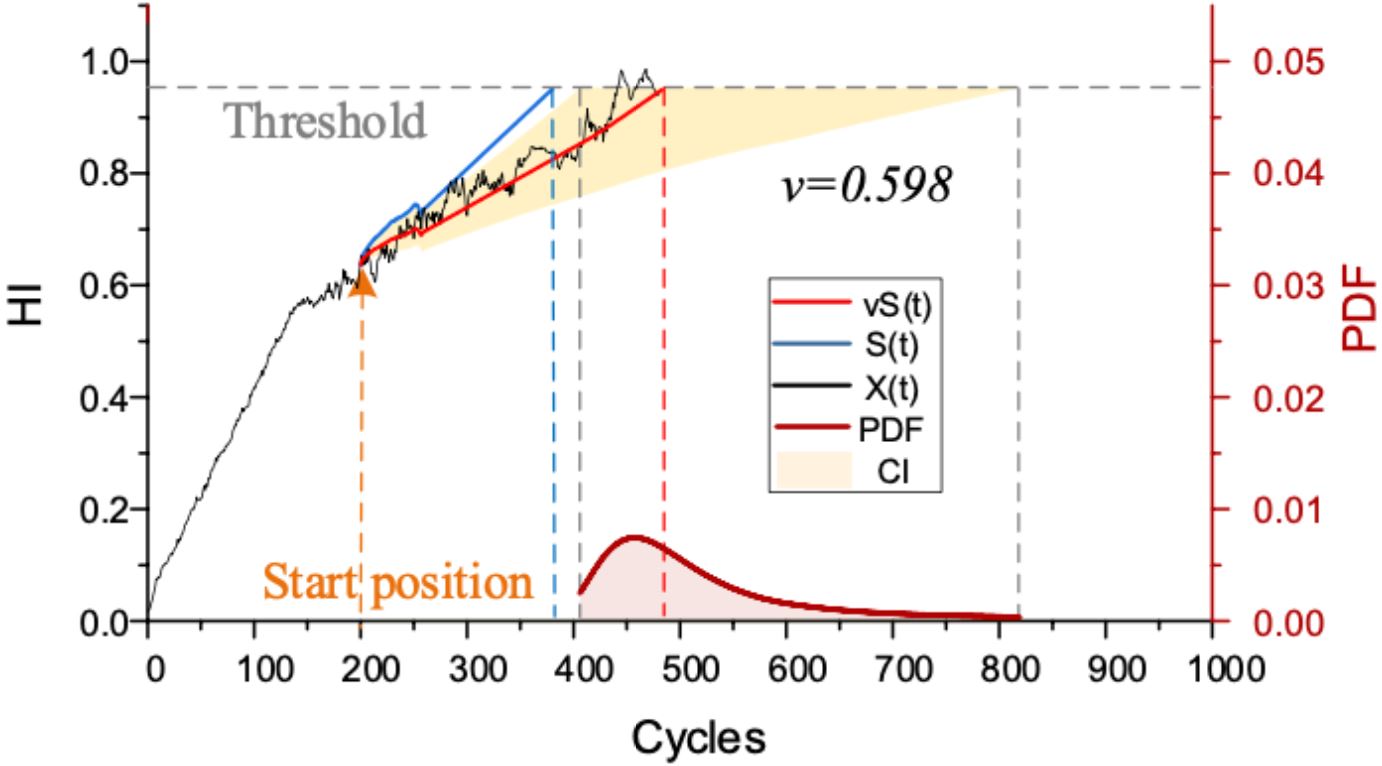
Estimating remaining useful life
One of the ways to plan for maintenance requirements is to determine the remaining useful life of assets. However, uncertainty dictates that predictions further into the future will be less precise.
Use a hybrid statistical and machine learning approach to improve the prediction of remaining life.
Upcoming courses
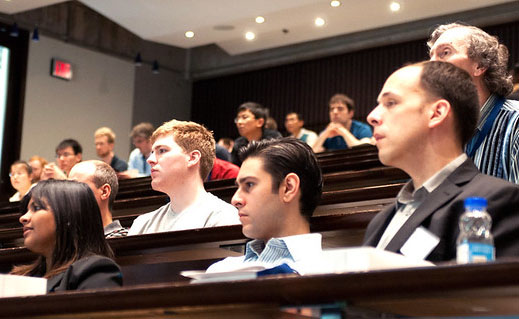
Physical Asset Management Nov 13-17, 2023 Registration is now open!
C-MORE has an exciting new addition to our professional development offerings! Our new 3531 Physical Asset Management course is a 5-day course focusing helping managers care for company assets and achieve maintenance excellence.
It is offered through the University of Toronto School of Continuing Studies. Register and find out more here.
Keep in touch!
Join our mailing list to stay informed on our latest activities